From Voices to Vulnerabilities: How OSINT Can Help
- Emerald Sage
- Nov 28, 2023
- 7 min read
Updated: Dec 8, 2023
On 18 February 2022, a news website released a video of Ukrainian President Volodymyr Zelensky declaring an end to the ongoing conflict against Russia. He stated Ukraine would surrender.
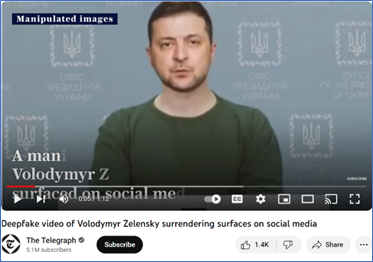
Shortly after this video was released, it was reported as fake. Analysts referred to the President’s behaviour as uncharacteristic. There were certain visual cues which made watchers question the authenticity of the footage. While the person in the video looked like the president, facial movements seemed slightly out of sync with the movements of the rest of his head, suggesting the face had been superimposed on a pre-existing image. Furthermore, Volodymyr Zelensky himself stated the news footage was fake in a video uploaded to his official social media channels. He stated the only people who would surrender would be Russian armed forces in Ukraine.
Videos like the footage of Volodymyr Zelensky surrendering, are becoming easier to produce with publicly available tools using a class of artificial intelligence technologies called generative AI. This technology generates realistic video content through deep learning models, such as Generative Adversarial Networks (GANs). These models, consisting of a generator and a discriminator, can create new video content, from a training data set. The discriminator distinguishes between real video frames from the training dataset and the fake ones created by the generator. The discriminator quickly trains the generator to create more accurate and realistic content through continuous feedback. The implication is continuous improvement. If the Volodymyr Zelensky video was generated today, it would probably look more coherent and realistic.
Where the Pressing Vulnerabilities Lie
While innovations in generative AI have made it easier to create more realistic visual content, we can apply analytical techniques to images which allow us to quickly make inferences about authenticity. When we analyse an image, we can look at elements like light and contrast and made inferences about whether shadows are consistent with the direction of the light source in the image. We can look at patterns and unity – specifically whether there are any disruptions to patterns, forms, colours, or shapes that shouldn’t be there. In video footage we can analyse movement and behaviour and make inferences about whether the subject or situation looks typical or consistent. Essentially, fake visual content, although easy to produce, can generally still be detected by the human eye.
However, audio content created from generative AI enabled tools can be much harder to detect. Audio content doesn’t possess the features and characteristics which allow the human eye to quickly detect anomalies. And voice cloning, or the use of machine learning algorithms and AI techniques to replicate and synthesise an individual's voice, have come a long way. As early as 2016, Google and Baidu had released Text to Speech (TTS) tools which could produce voices which sounded human. Since then, these tools have been supplemented and refined by learning systems like neural networks which can decode the intricate network of sound and convert it into sophisticated representations. GANs are also used to improve cloning by refining fake voices to make them sound more like a real person. Together, neural networks and GANs enable the replication of the speaker's vocal characteristics, including speech patterns and intonations.
We are therefore particularly vulnerable to generative AI created audio content used for malicious purposes. To date, the harmful application of this technology has included the targeting of individuals and businesses with fraudulent extortion scams. Creative professionals and public figures have also had their voices appropriated in ways that threaten their livelihoods and deceived the public.
For example, multiple media outlets reported last month that an audio clip uploaded to social media in the lead up to the Slovakia National elections, featured a voice that sounded like the country’s Progressive Party leader, Michal Šimečka. In the audio clip Michal Šimečka can be heard describing a scheme to manipulate the national vote by bribing members of the country’s marginalised Roma population. Although fact checking by local media outlets ultimately found this clip to be fake, some users who engaged with the associated online content, appear to have believed it.
Where OSINT Tools and Techniques Can Help
Open-source intelligence (OSINT) is generated by collecting and analysing publicly available information to address a specific question. To analyse publicly available information in an online environment where generative AI enabled content can be used to deceive, there is a need to carefully evaluate information sources. We can do this using a variety of free and publicly available OSINT tools and techniques.
Analytical Techniques
There are a range of techniques or methodologies we can use to evaluate information sources. An example is R2-C2 which frames an evaluation around relevance, reliability, credibility and corroboration. For example, when applying this methodology to the evaluation of the audio clip of Michal Šimečka we could:
Assess the information for relevance which involves asking questions like - What is the audio clip trying to achieve? What is being said, who is saying it and what does the timing of the clip’s release tell us about the intent for releasing it? Who or what would benefit from a Progressive Party defeat in the Slovakia elections?
Assess the reliability of the source of the information which involves asking questions like - Who are the people or organisations who have published the clip online? What are their political ideologies and affiliations?
Assess the credibility of the information, which involves reflecting on what we know about Michal Šimečka. Is he likely to engage in election fraud? Is this consistent with what we know about him? Does he have links that we know about to the Roma population in Slovakia?
Corroborate this information with other sources by ascertaining whether reputable news outlets with fact checking capabilities are reporting this audio clip as fake or genuine?
Evaluation methodologies provide us with a cognitive framework for authenticating information. We can support this framework with a range of free and publicly available tools.
Fact Checking Tools
Fact-checking tools are online resources which seek to verify the accuracy and validity of claims, statements, or information. These tools utilise various methods, such as cross-referencing information with reliable sources, checking data against databases of known disinformation, and/or incorporating human analysis of any associated evidence like images.
Google Fact Check Explorer is a free fact checking tool with a search field that allows users to input a name or topic and retrieve any online content reported as false about that name or topic. Input can be configured like a google search, making it possible to filter by parameters like language or publisher.

Results will typically contain the originator, a snapshot of the information where it is relevant to the search criteria, the evaluation rating which can include summaries like “False” or “Edited Video”. Importantly, Google’s Fact Check Explore will also provide the source of the evaluation rating, so a user can check Google’s verification manually.

Other fact checking tools include: FactCheck.org which claims to advocate for voters by reducing the level of deception and confusion in politics; and Snopes which claims to focus on, but is not limited to, validating urban legends in American popular culture.
Behavioural Analysis Tools
When evaluating information, we can use tools to analyse the information posted online and evaluate the profiles used to upload and propagate this information. As early as 2017, researchers at the University of Edinburgh's School of Informatics, had analysed large social networks for common behaviours that characterised fake profiles. This research led to the design of freely available tools that automate the detection of the behaviours identified in this study and assign posts and profiles an authenticity rating.
FollowerAudit is an example of a behavioural analysis tool for Twitter and Instagram profiles. It features a search field where users can input an account and analyse associated followers using features such as social network structure, temporal activity, language, and sentiment. It requires an account to analyse profiles and will seek authentication to use a registered account before returning results.
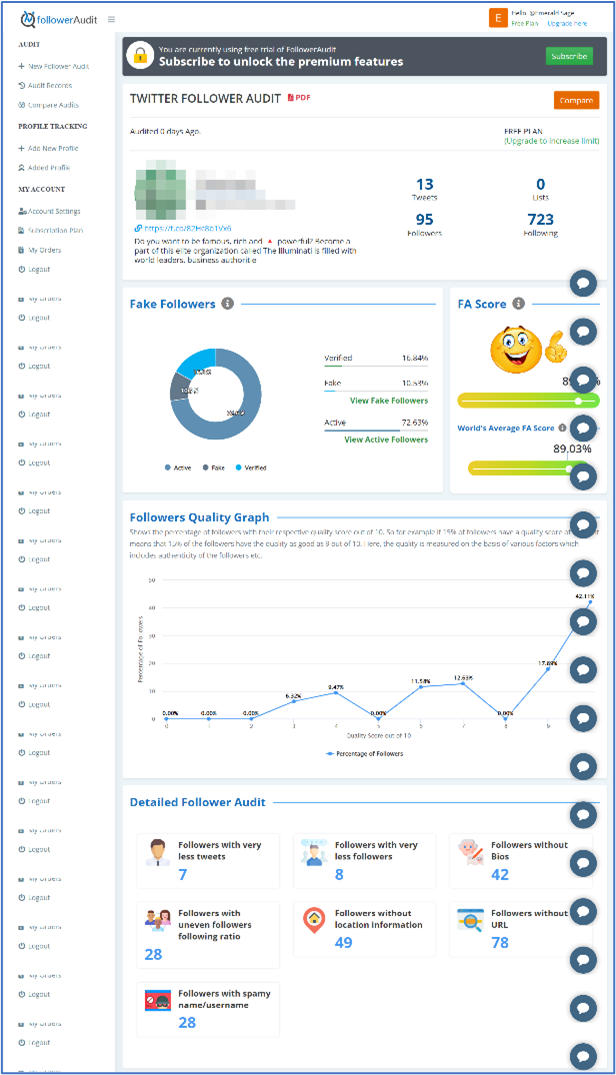
Spectral Analysis Tools
Spectral analysis is a technique used in signal processing to understand the ‘spectral characteristics’ of a signal - or the unique properties or features of a signal when it is represented in the frequency domain. It involves breaking down a complex signal into its individual frequency components to study their presence, amplitude, and relationship. Voice cloning can introduce subtle discrepancies in the spectral characteristics of the cloned voice, such as alterations in pitch, resonance, or harmonics. Spectral analysis can potentially detect these anomalies by comparing the spectral profile of the cloned voice to that of the original speaker.
Audacity is a free to download multi-track audio editor and recorder that can display voice tracks in a spectrogram. This means it can render a graphical representation of the spectrum of frequencies in a signal as they vary with time. It allows users to upload and analyse an original voice track and then compare against a generative AI cloned voice track. When we performed this exercise, we found some differences in the intensity of colour at points within the spectrogram, indicating the magnitude or amplitude of the frequency components at a specific point in time differed across both recordings (see below).


However, it's important to note that voice cloning technology is becoming increasingly sophisticated. Cloned voices are often difficult to distinguish using spectral analysis alone. It’s important that we use a range of tools and techniques when analysing the authenticity of audio content. In addition to cognitive frameworks like R2-C2, fact checkers, and behavioural analysis tools, we can also employ professional linguistic, and voice technology services, particularly in situations where our assessments may impact life or liberty.
Overall, being aware of the OSINT tools and techniques we can use to help verify the authenticity of audio content is vitally important, particularly given how difficult it can be to detect fake audio with the human ear alone. When we combine this awareness with the ability to identify and articulate the vulnerabilities that malicious actors can exploit, we can take measures to safeguard our digital landscape.
If you would like to dive deeper into using Generative AI for OSINT, be sure to look at our training offerings in 2024, or contact us to see how we have integrated AI into our flagship product, NexusXplore.
Comments